機械学習の相互運用性のためのオープンスタンダード
Inferenceノードからサードパーティの機械学習トレーニング済みモデルをインポートしします。
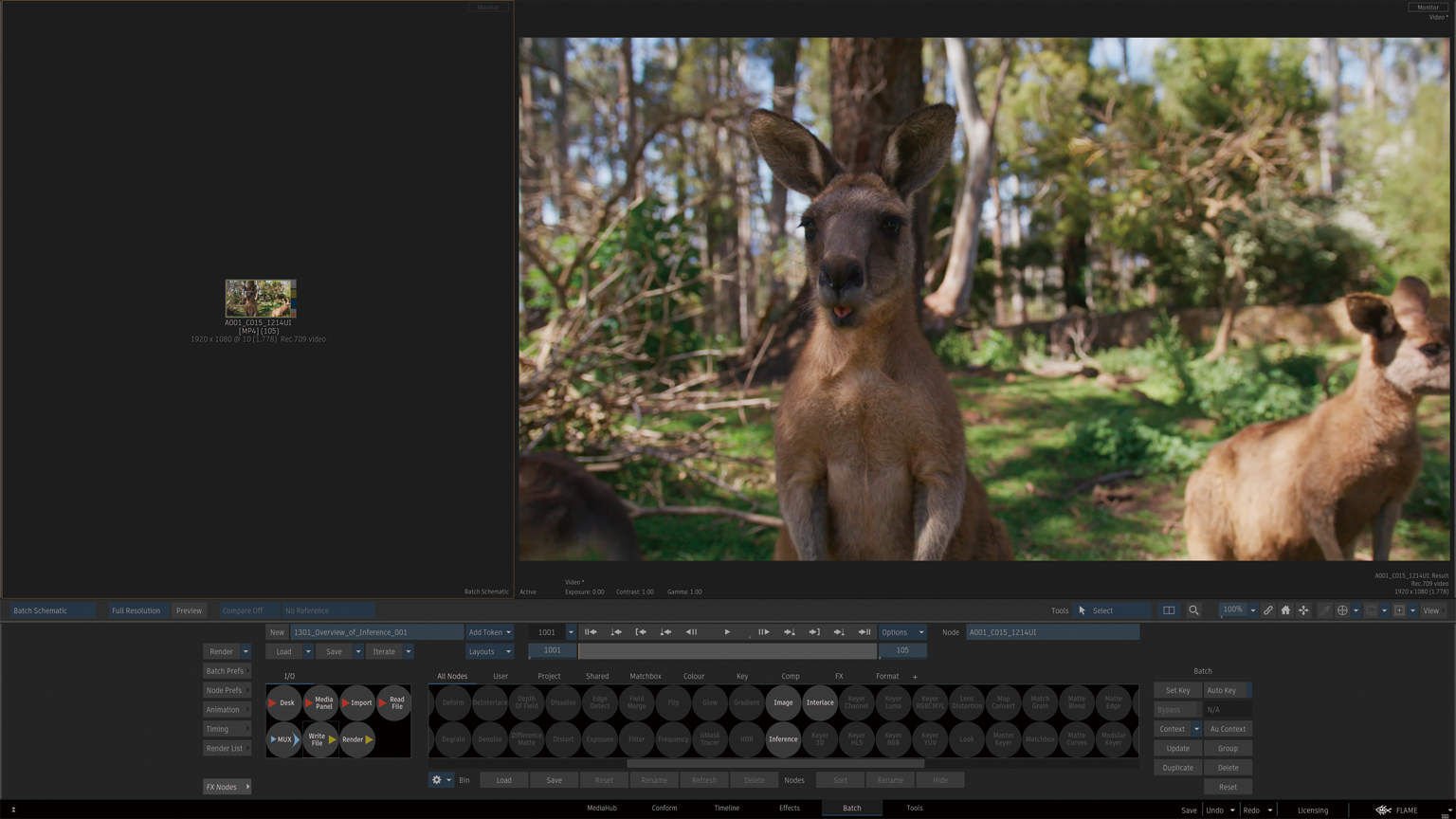
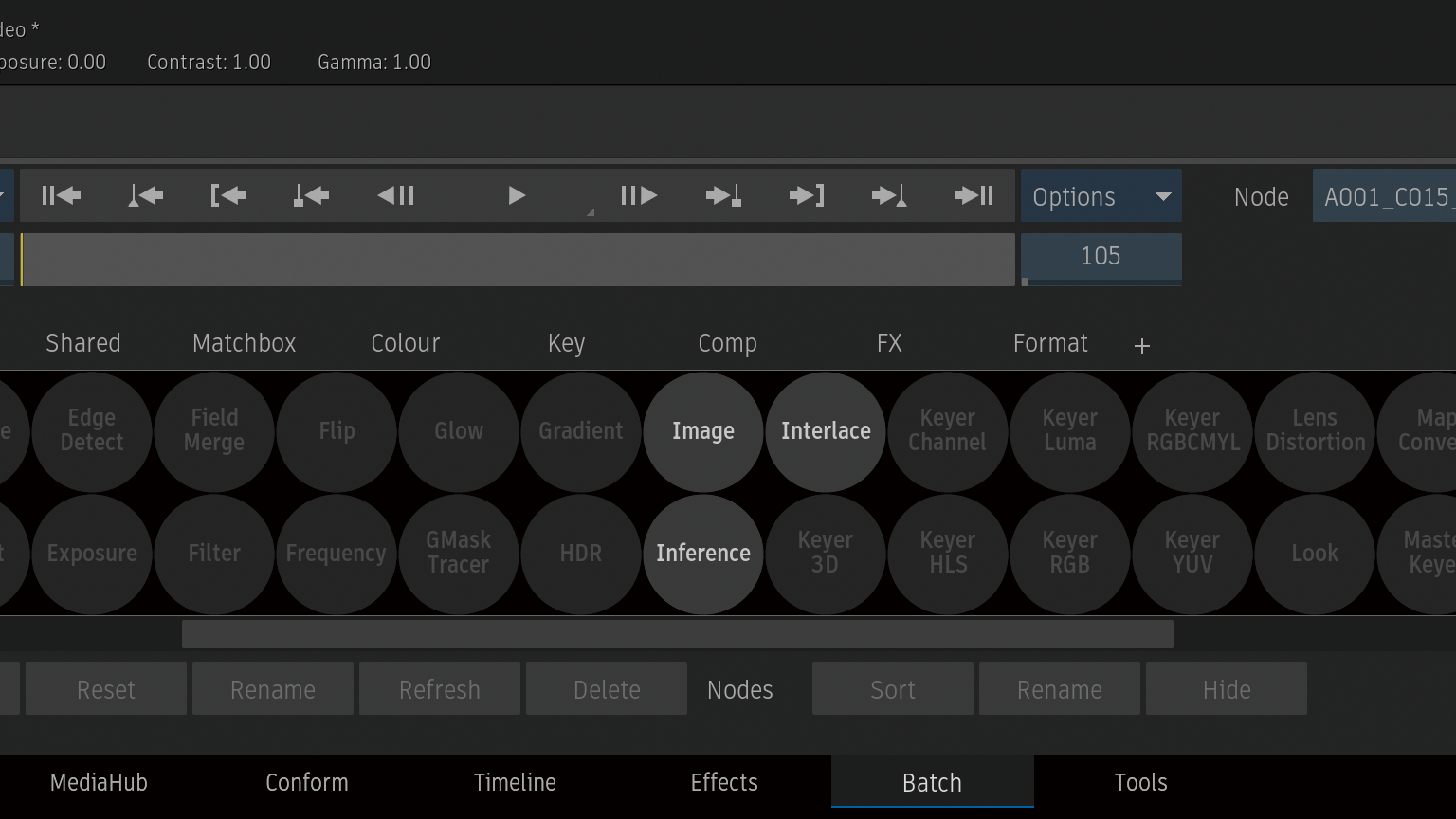
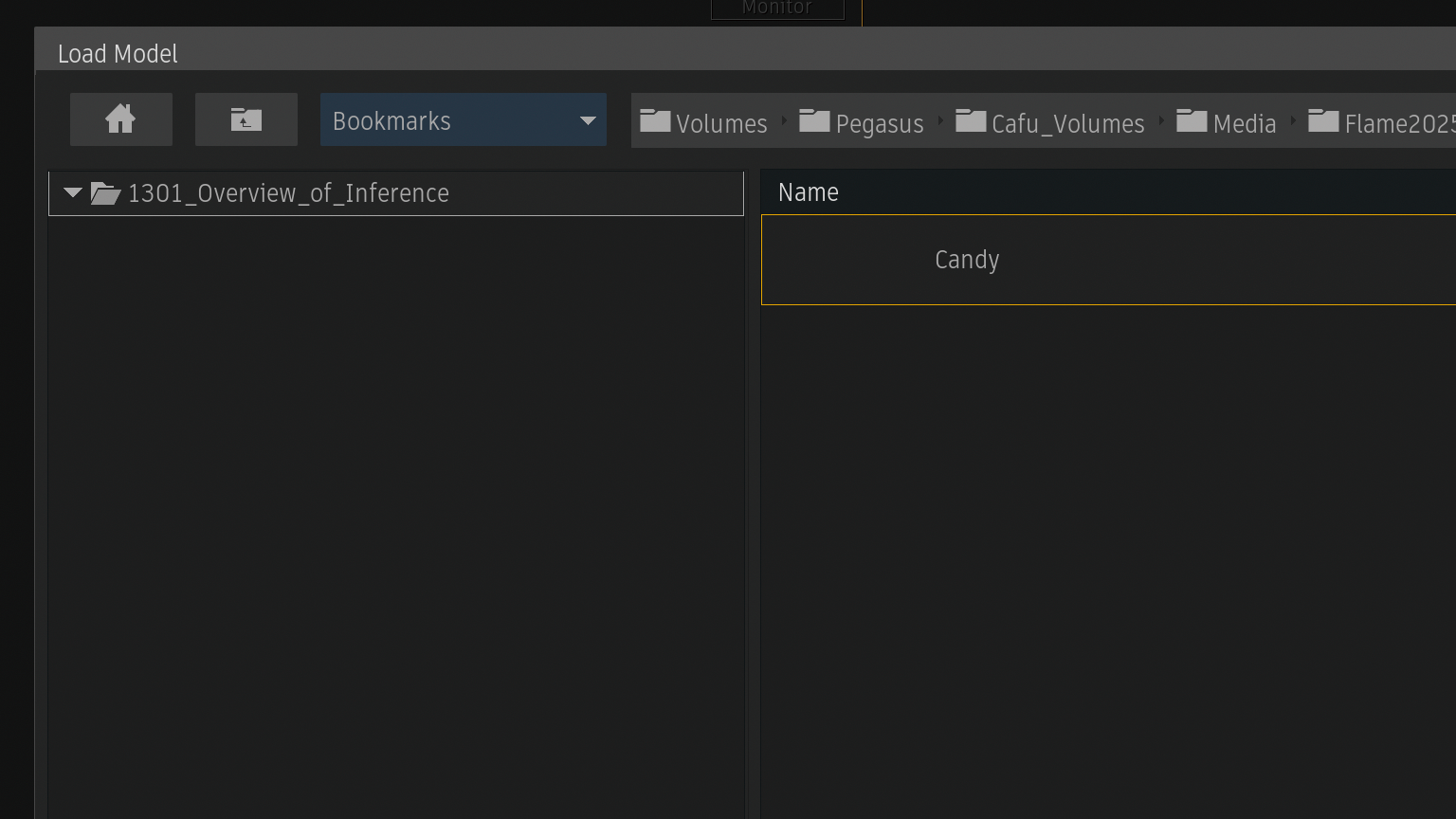
ロードできるファイルは、.onfファイルと.onnxファイルの2種類です。
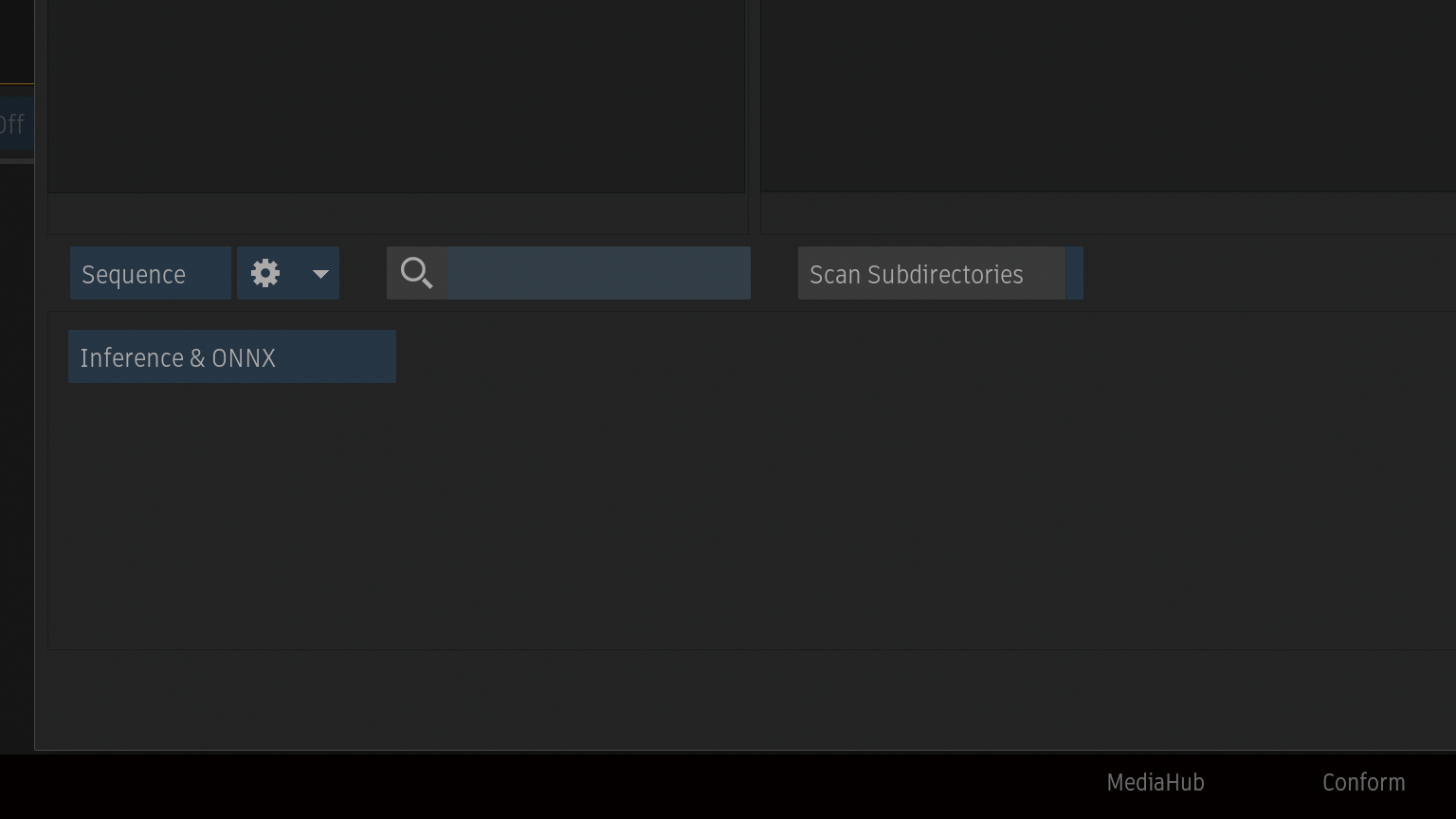
.onnxファイルを選択すると、ソフトウェアはディレクトリ内で同じ名前の .jsonファイルを検索します。その.jsonファイルは、モデルの入力、出力、および属性を定義します。これは、Matchboxシェーダーの.xmlファイルに相当します。関連する.jsonサイドカーファイルが同じディレクトリに存在しない場合、アプリケーションはデフォルトの入力、出力、および属性をノードに適用します。
.onnxモデルをそのままInferenceノードにインポートすることもできますが、一部のモデルでは正しく動作させる前に特定の設定を調整する必要があります。
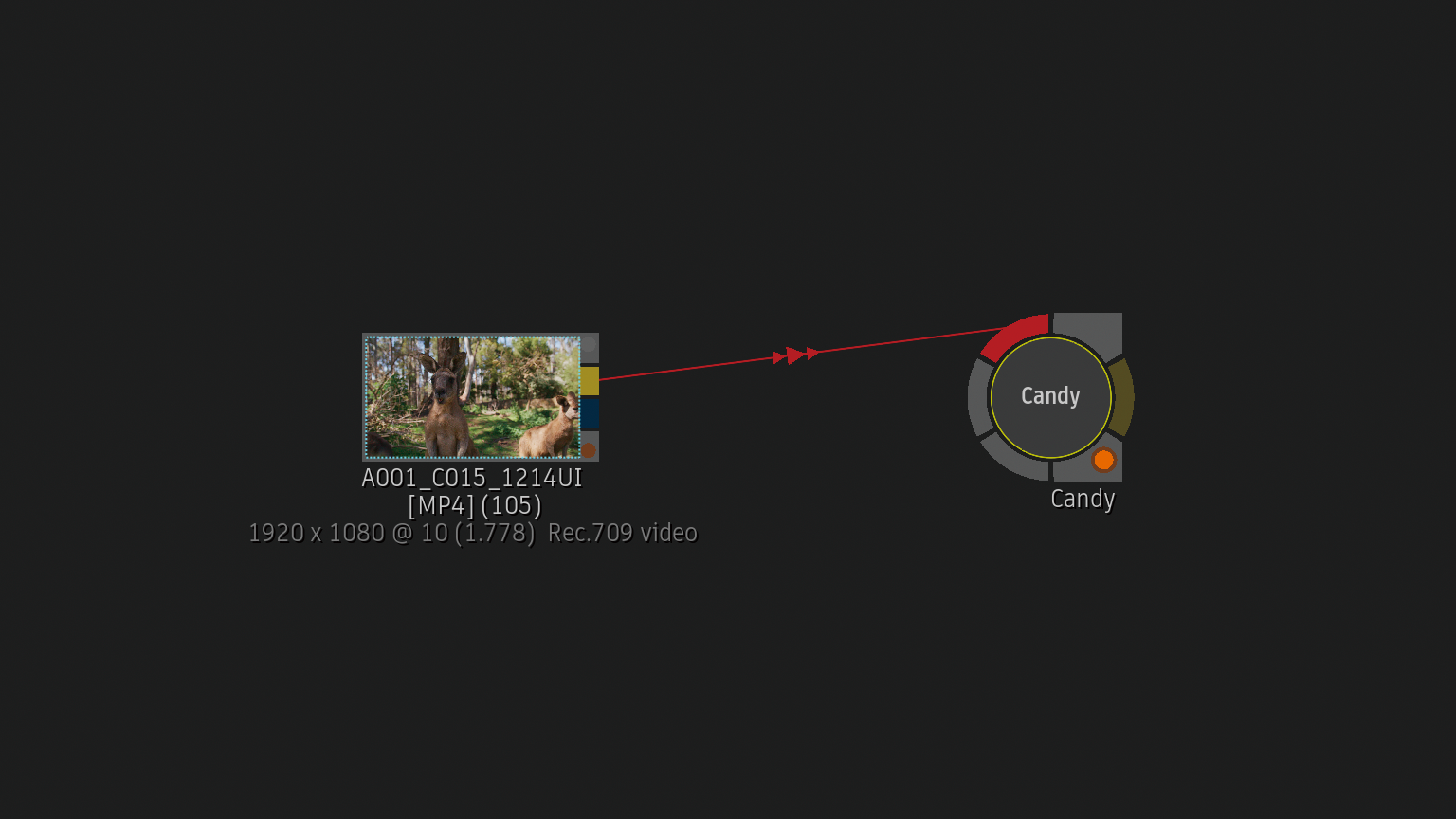
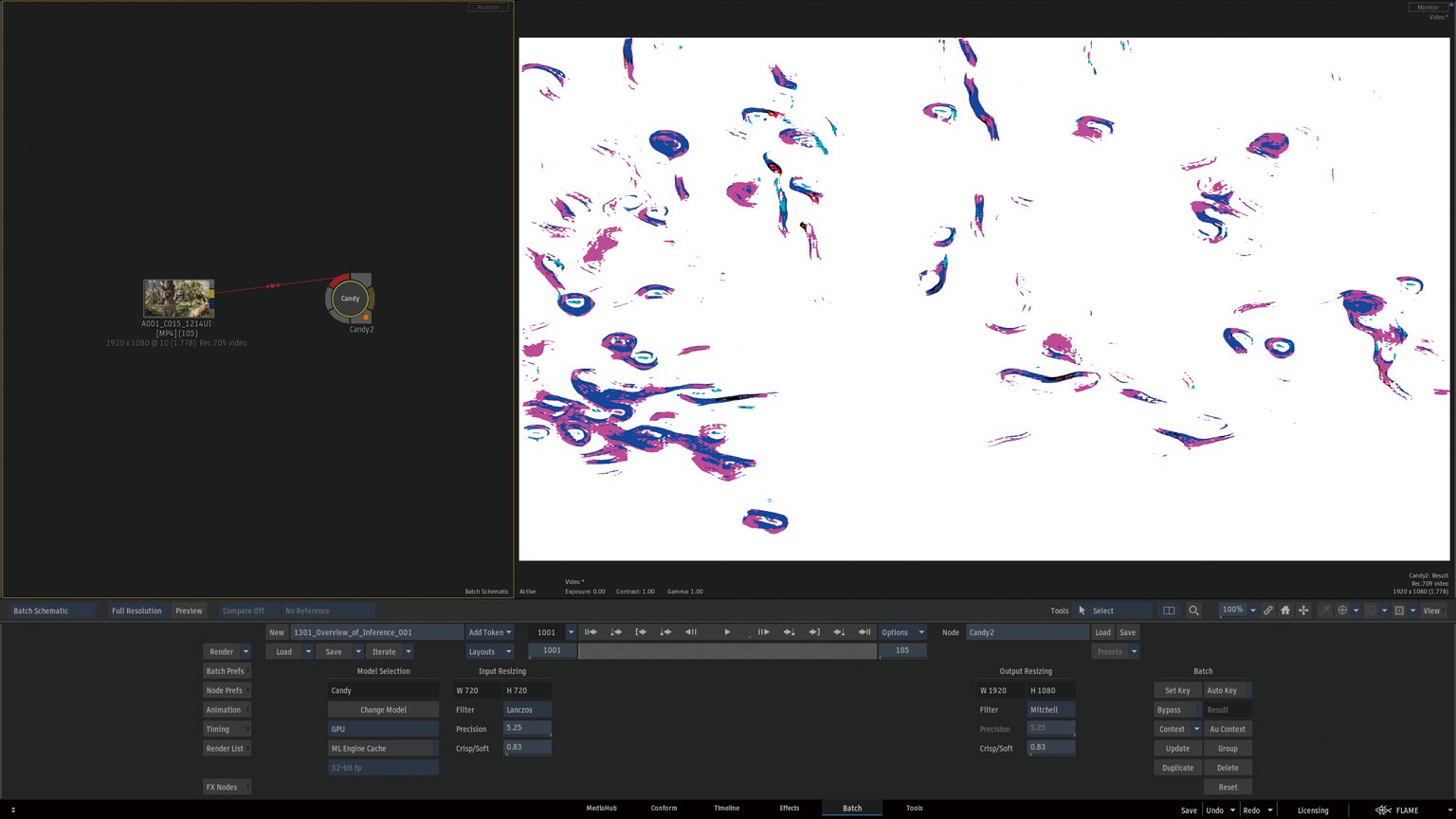
このモデルは0 – 1ではなく0 – 255範囲の符号なし整数でトレーニングされているので、Flameは入力に255のGainを適用し、出力に255の逆ゲインに変更する必要があります。
Figo:~ admin$ /opt/Autodesk/flame_2025.2.2/bin/inference_builder
Usage : inference_builder [OPTION...] ONNX FILE
-p, --package creates a '.inf' package file
-u, --unpack extracts the JSON and thumbnail (.png) files from an '.inf' package file
-j, --write-json writes the ONNX model description in a JSON file
-o, --output outputs all files in the specified folder
The destination folder must be specified using the following syntax:
-o <path>
--output=<path>
-h, --help displays the full documentation
Examples:
inference_builder -p foo.onnx
# creates an inference package
# (foo.inf) with the following files:
# foo.onnx, foo.json (if it exists) and
# foo.png (if it exists)
inference_builder -j foo.onnx # creates a JSON model description in foo.json
inference_builder -u foo.inf # extracts files foo.json and foo.png
Try `inference_builder --help' for more information.
Figo:~ admin$
-j オプションから.jsonサイドカーファイルを作成
/opt/Autodesk/flame_2025.2.2/bin/inference_builder -j /opt/Autodesk/shared/inference/models/Candy.onnx
generating JSON model description ...
creating JSON file (/opt/Autodesk/shared/inference/models/Candy.json) ... [OK]
Figo:1301_Overview_of_Inference admin$
.jsonのGainを修正
Figo:models admin$ cat Candy.json
{
"ModelDescription": {
"MinimumVersion": "2025.1",
"Name": "Candy",
"Description": "",
"SupportsSceneLinear": false,
"KeepAspectRatio": false,
"Padding": 1,
"Inputs": [
{
"Name": "inputImage",
"Description": "",
"Type": "Front",
"Channels": "RGB",
"Gain": 1.0
}
],
"Outputs": [
{
"Name": "outputImage",
"Description": "",
"Type": "Result",
"Channels": "RGB",
"InverseGain": 1.0,
"ScalingFactor": 1.0
}
]
}
}Figo:models admin$
Figo:models admin$ vi Candy.json
{
"ModelDescription": {
"MinimumVersion": "2025.1",
"Name": "Candy",
"Description": "",
"SupportsSceneLinear": false,
"KeepAspectRatio": false,
"Padding": 1,
"Inputs": [
{
"Name": "inputImage",
"Description": "",
"Type": "Front",
"Channels": "RGB",
"Gain": 255.0
}
],
"Outputs": [
{
"Name": "outputImage",
"Description": "",
"Type": "Result",
"Channels": "RGB",
"InverseGain": 255.0,
"ScalingFactor": 1.0
}
]
}
}Figo:models admin$
-p オプションからパッケージ化 (サムネールの.pngファイルは別途作成)
Figo:models admin$ /opt/Autodesk/flame_2025.2.2/bin/inference_builder -p /opt/Autodesk/shared/inference/models/Candy.onnx
using JSON file /opt/Autodesk/shared/inference/models/Candy.json ... [OK]
using thumbnail /opt/Autodesk/shared/inference/models/Candy.png ... [OK]
packaging /opt/Autodesk/shared/inference/models/Candy.inf ... [ OK ]
Figo:models admin$
Change Modelからロードモデルファイルブラウザーを表示
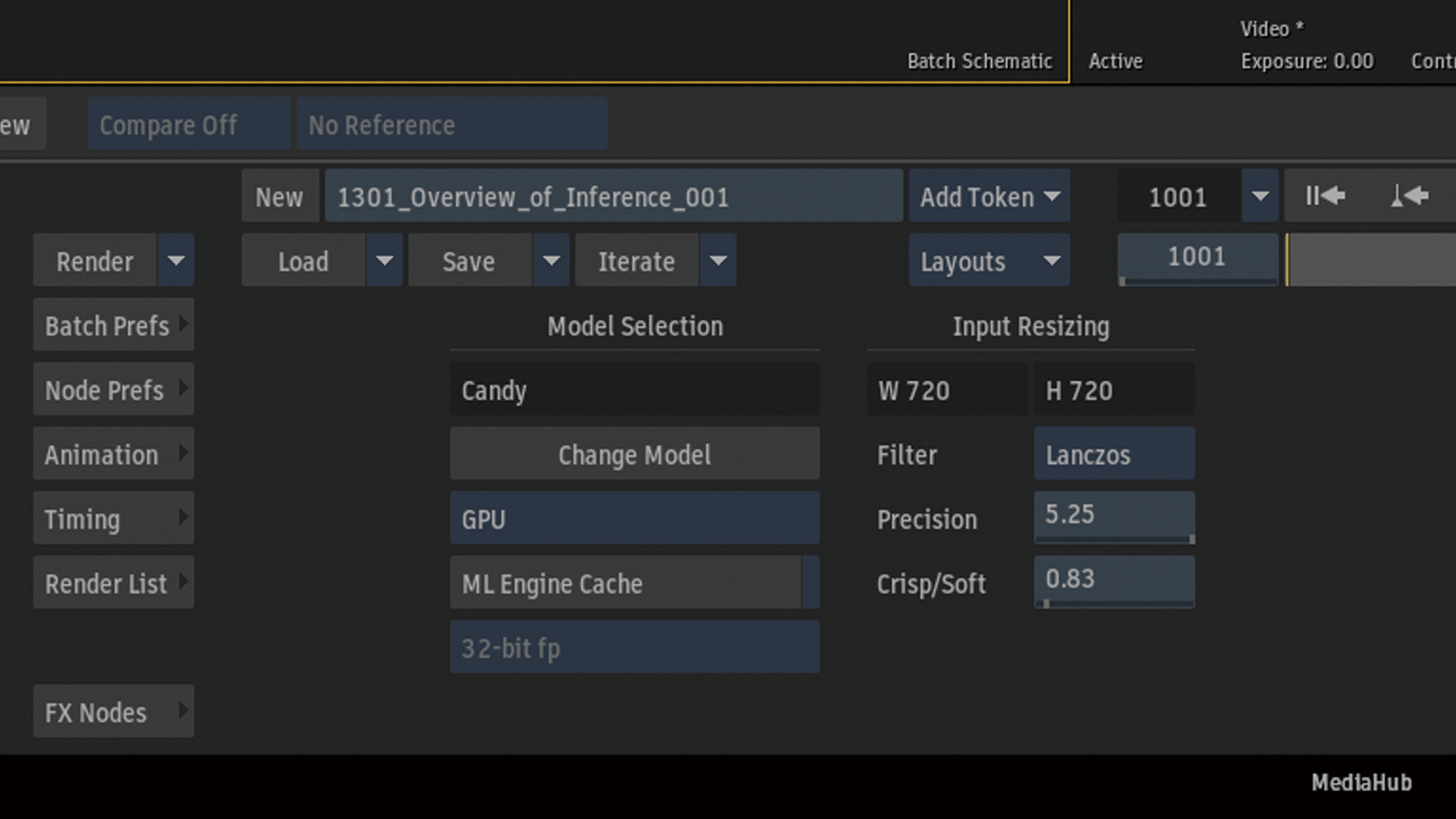
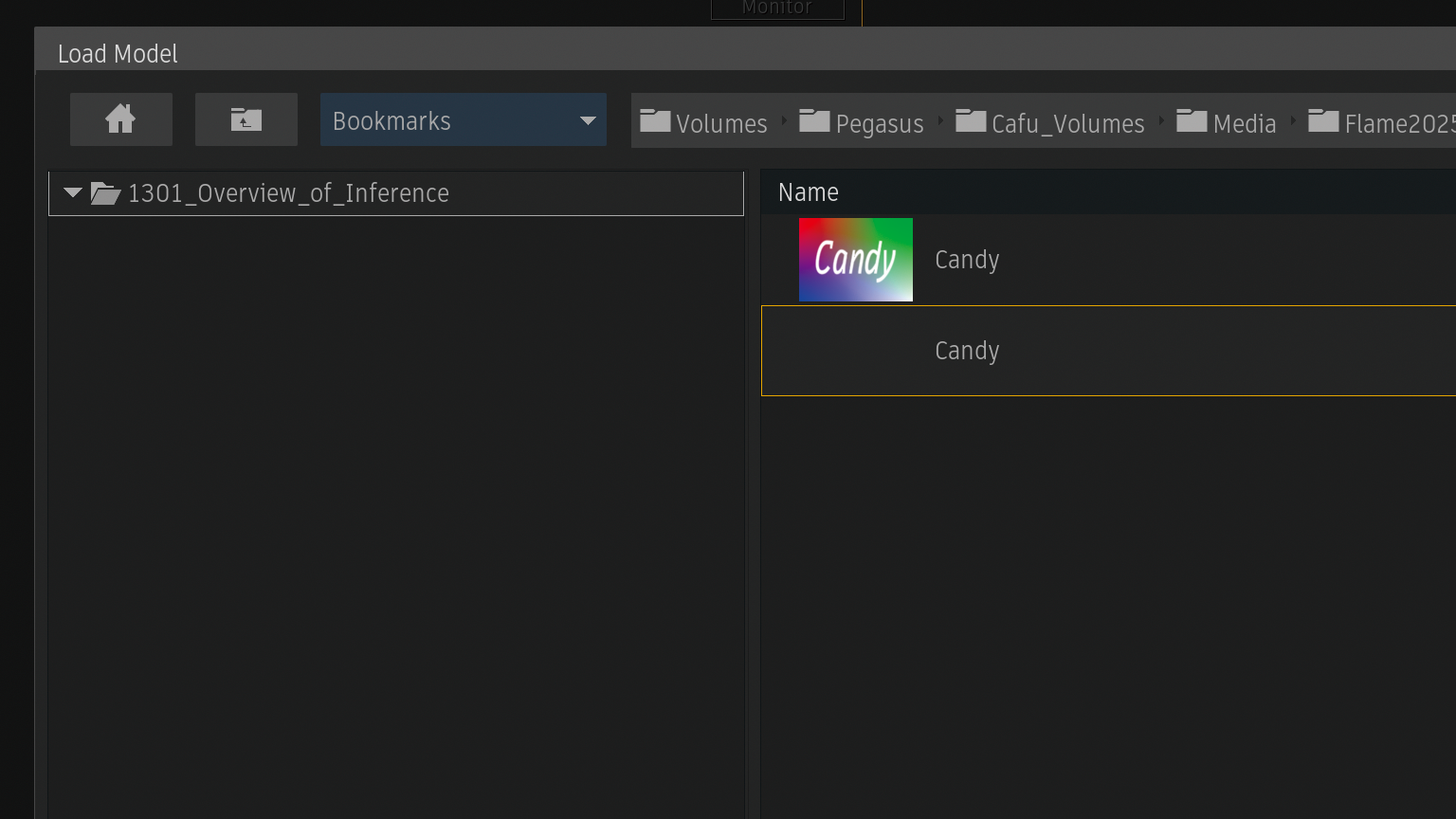
パッケージした.infファイルをロード
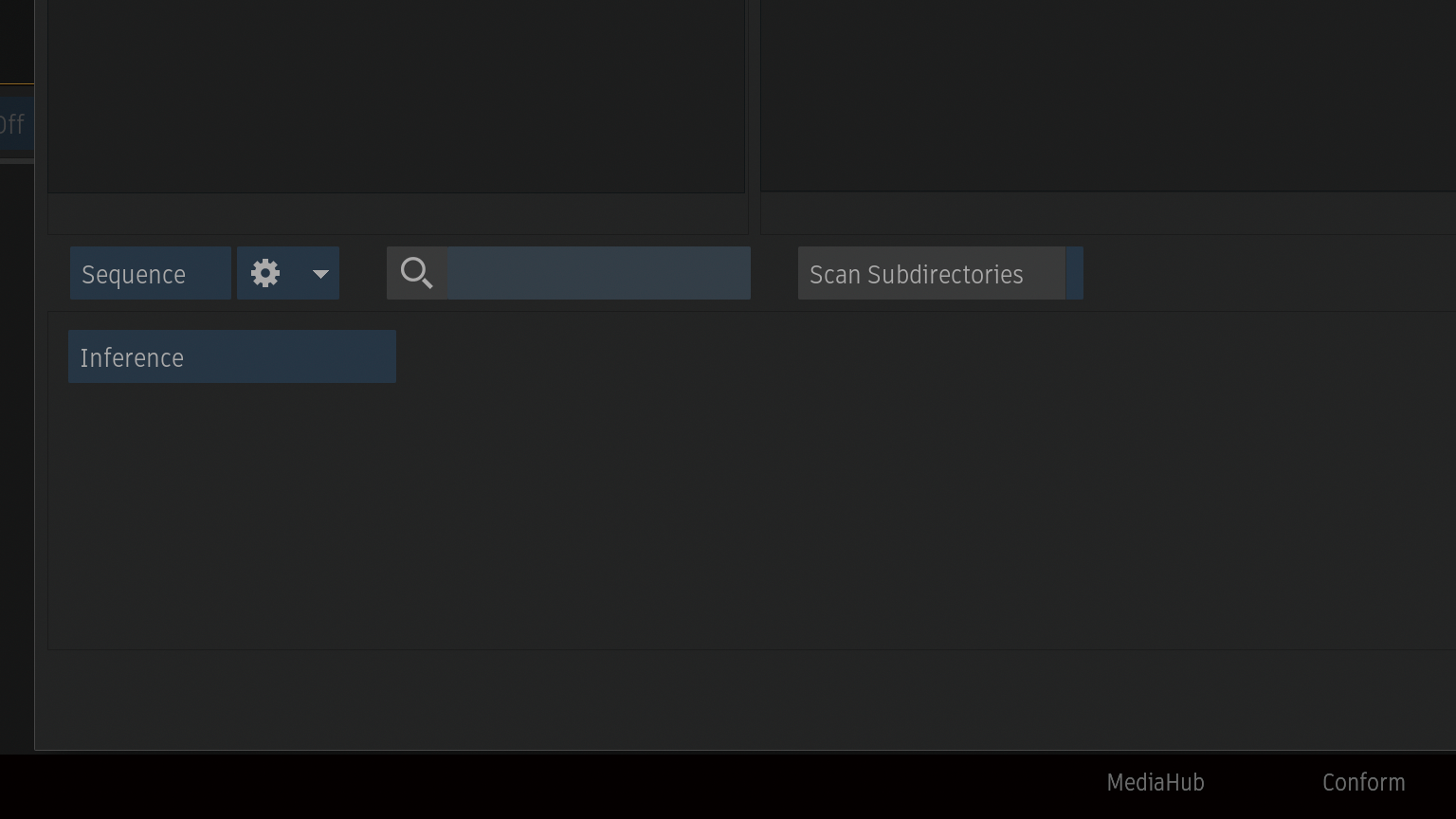
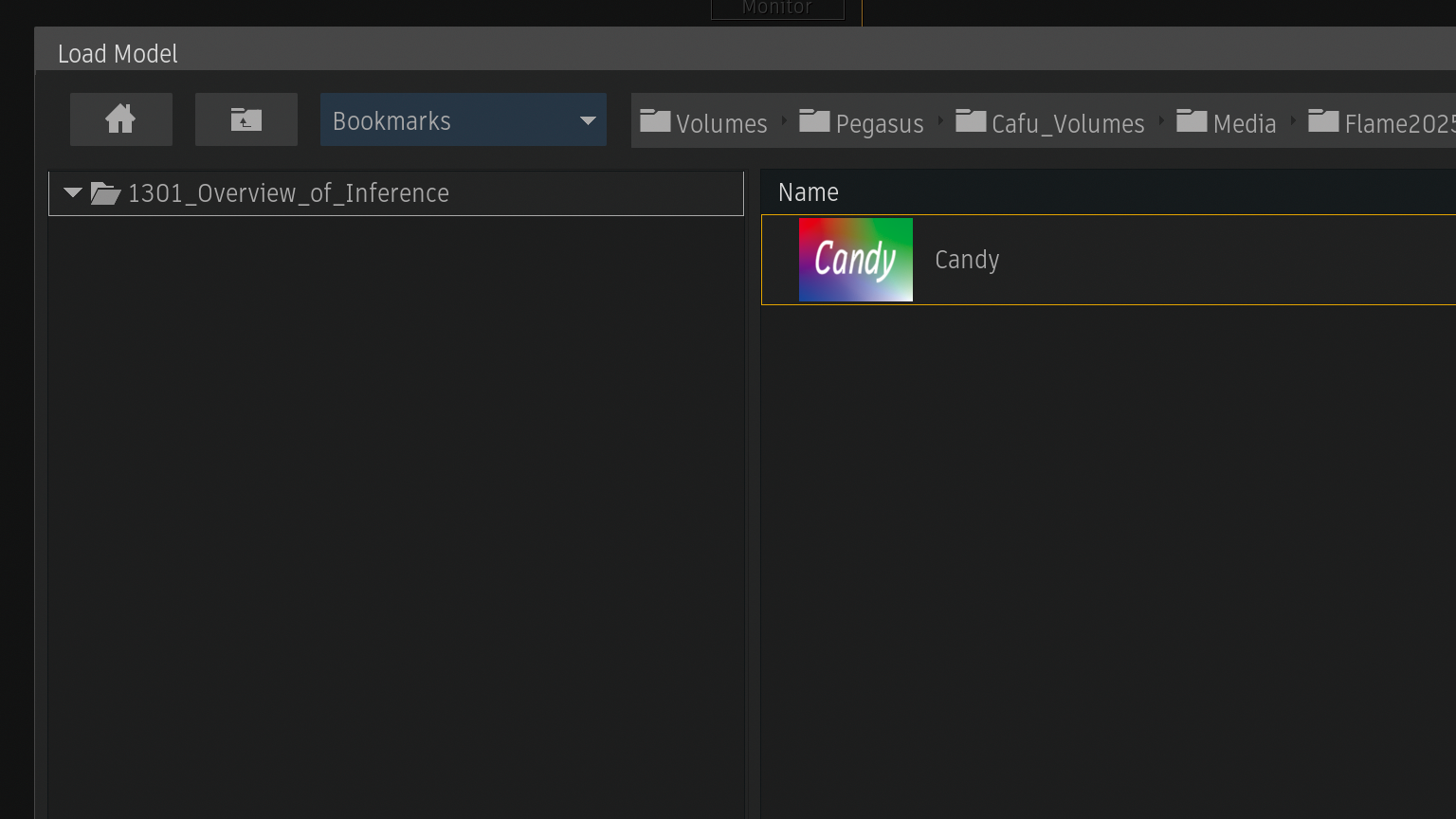
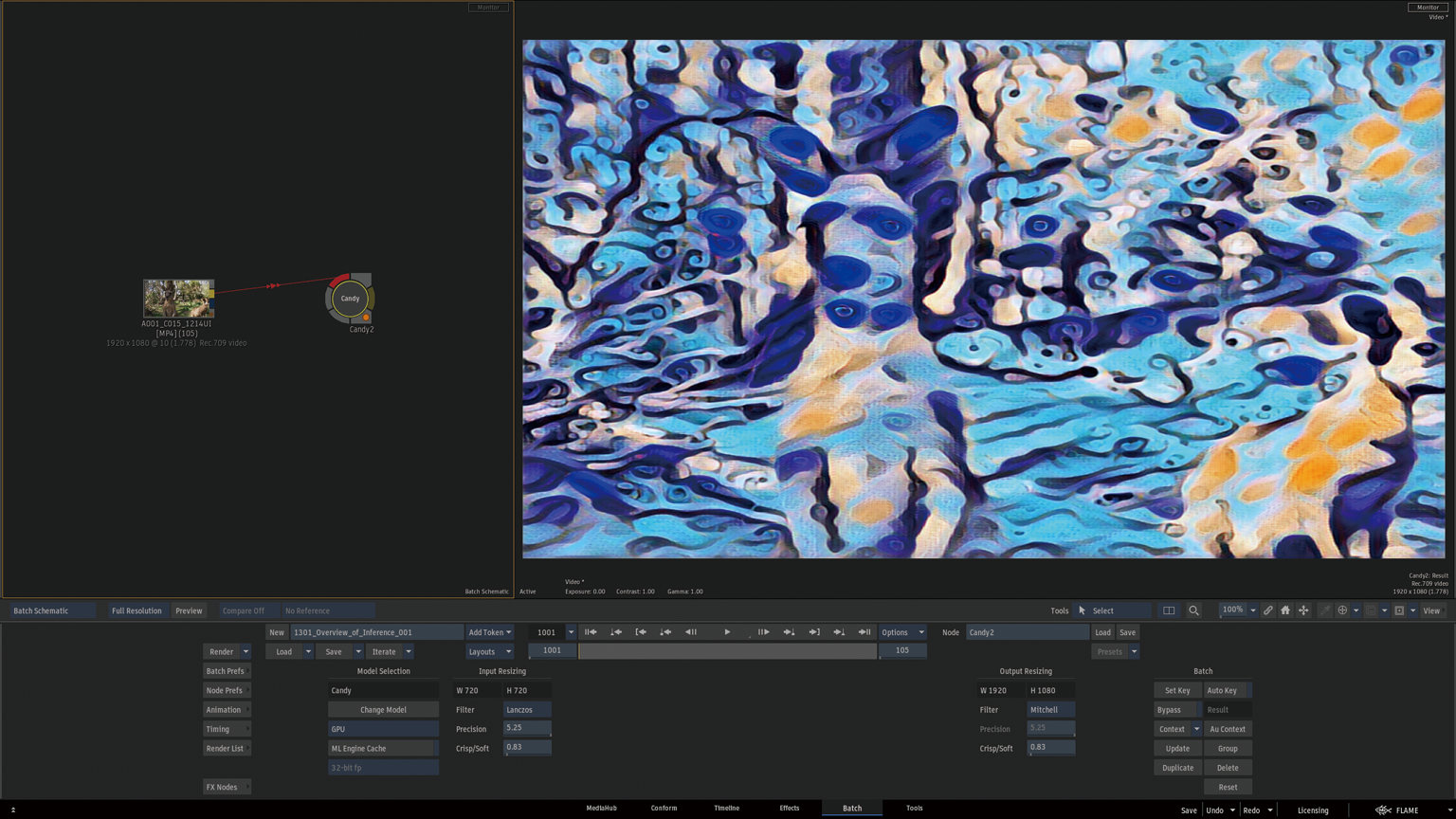
Rocky LinuxではGPUまたはCPUを選択することができますが、macOSではGPUを選択することができません。
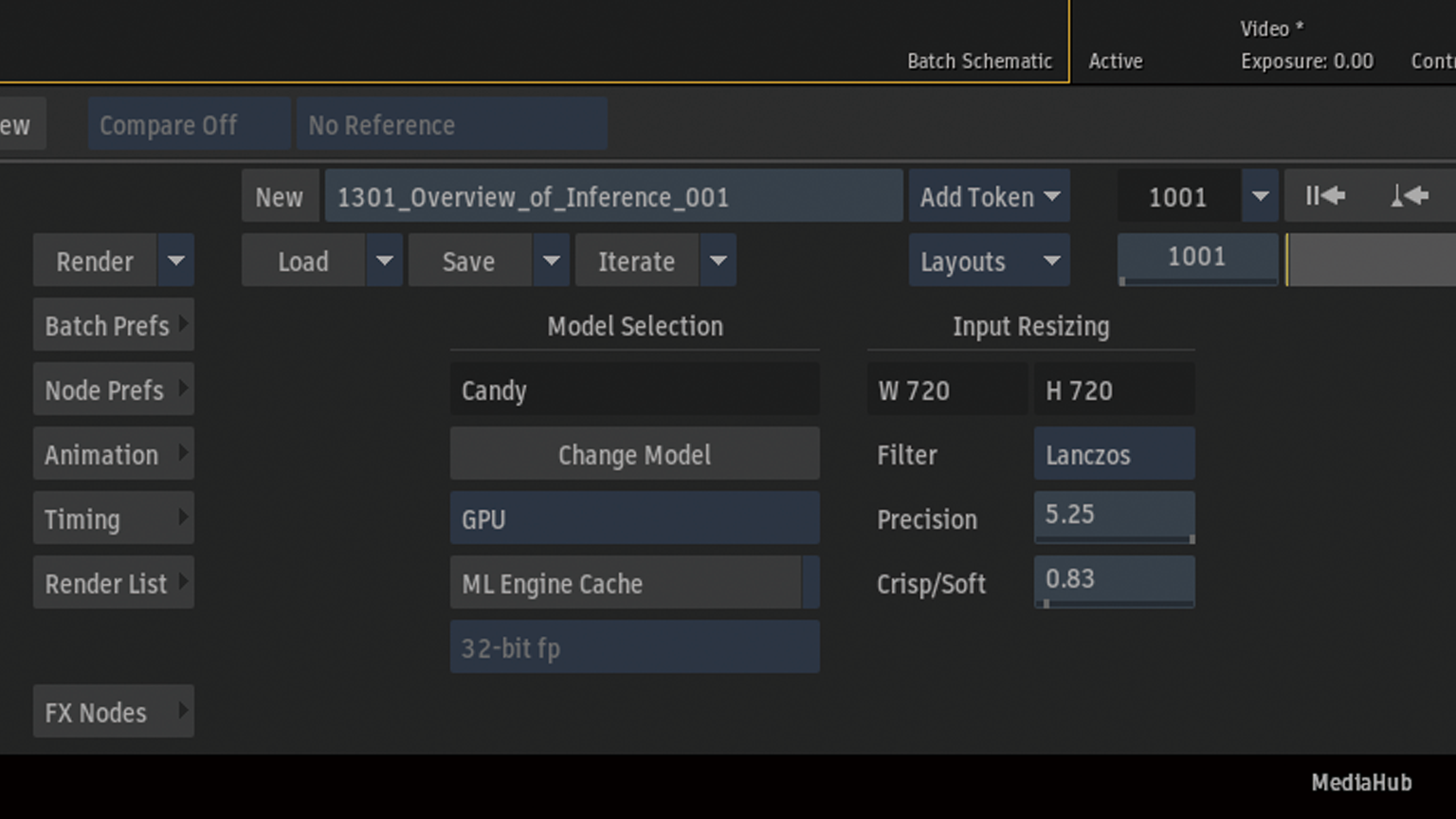
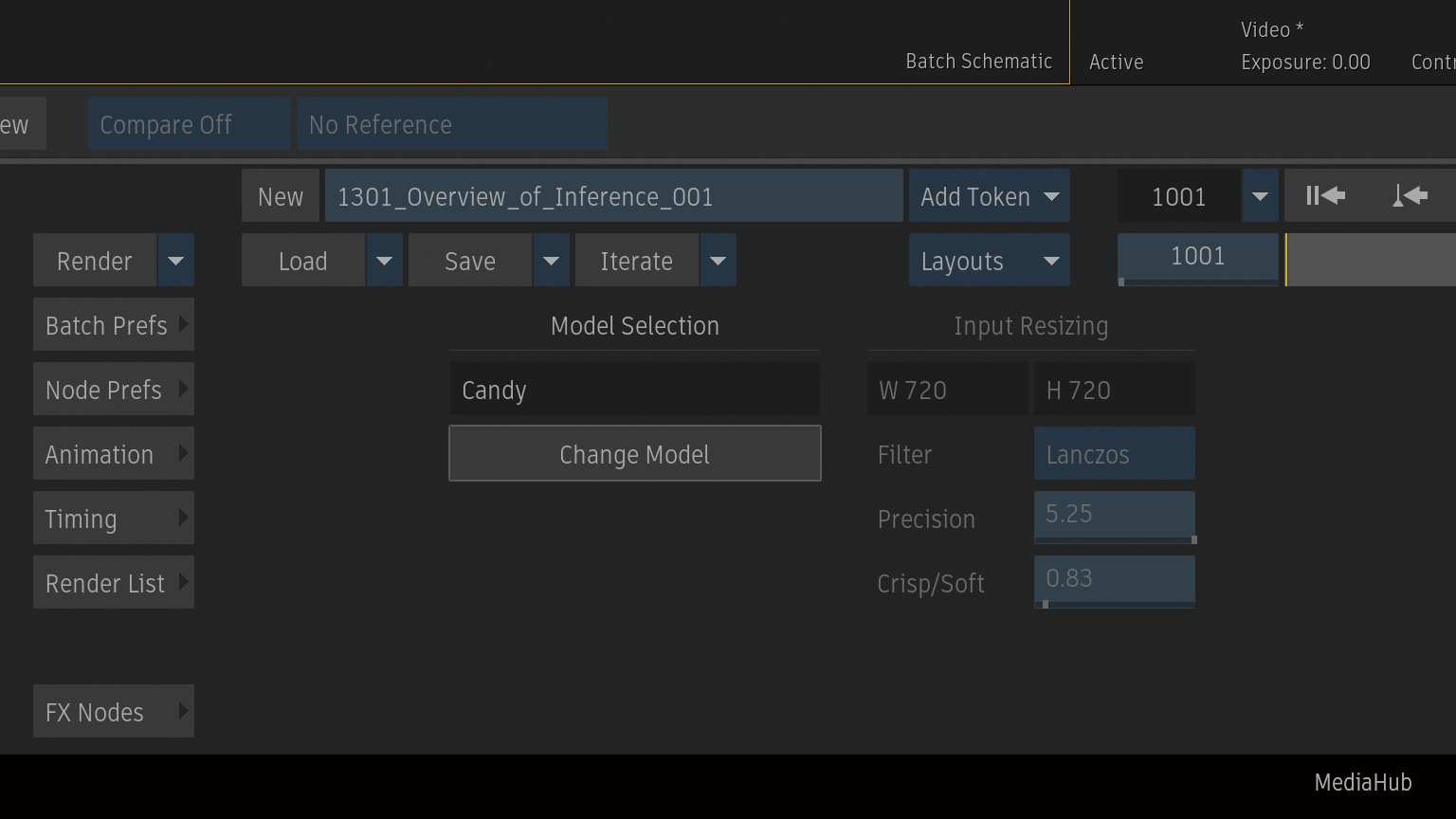
Rocky Linuxの場合、GPUとCPUを切り替えが可能です。
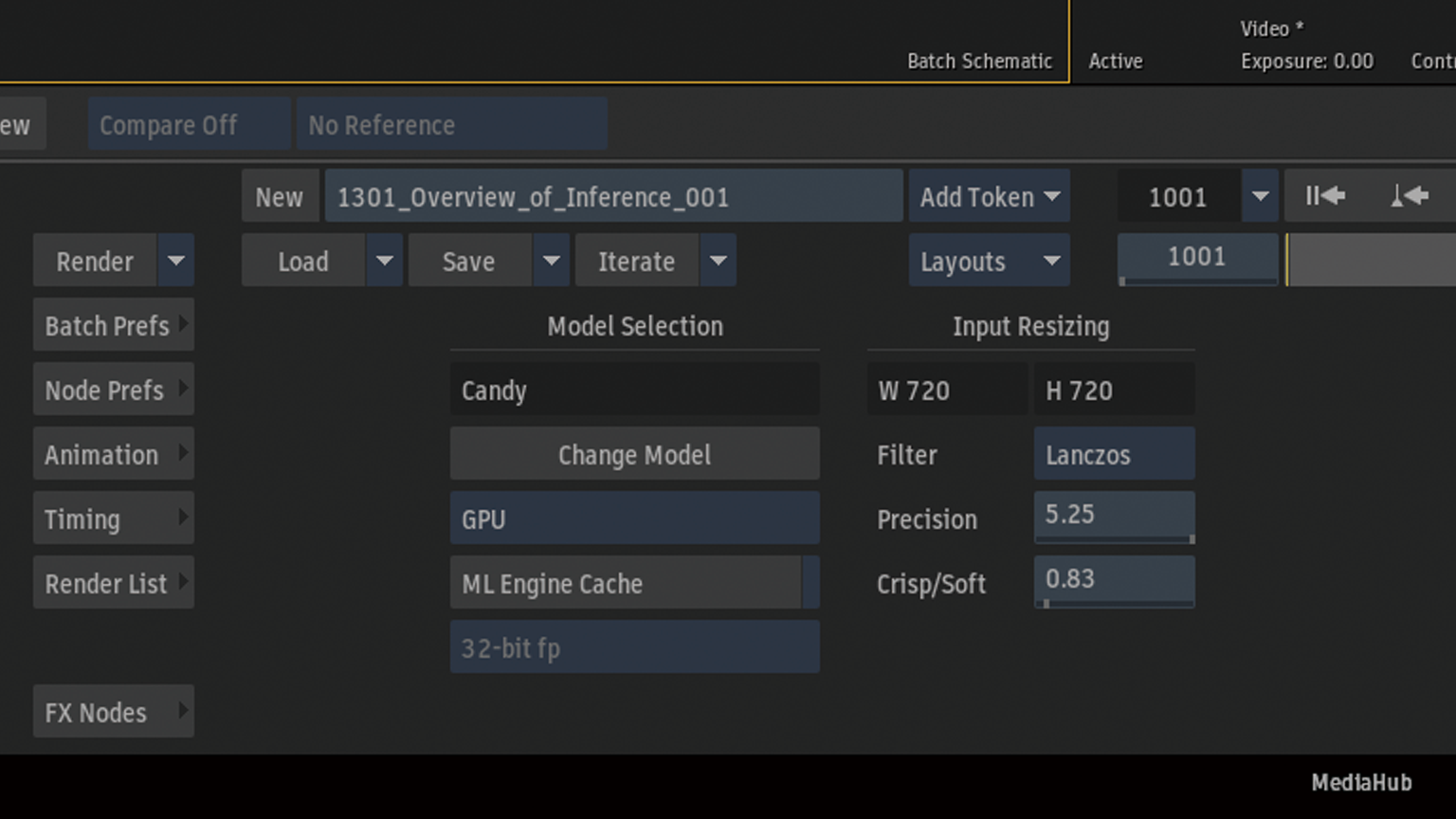
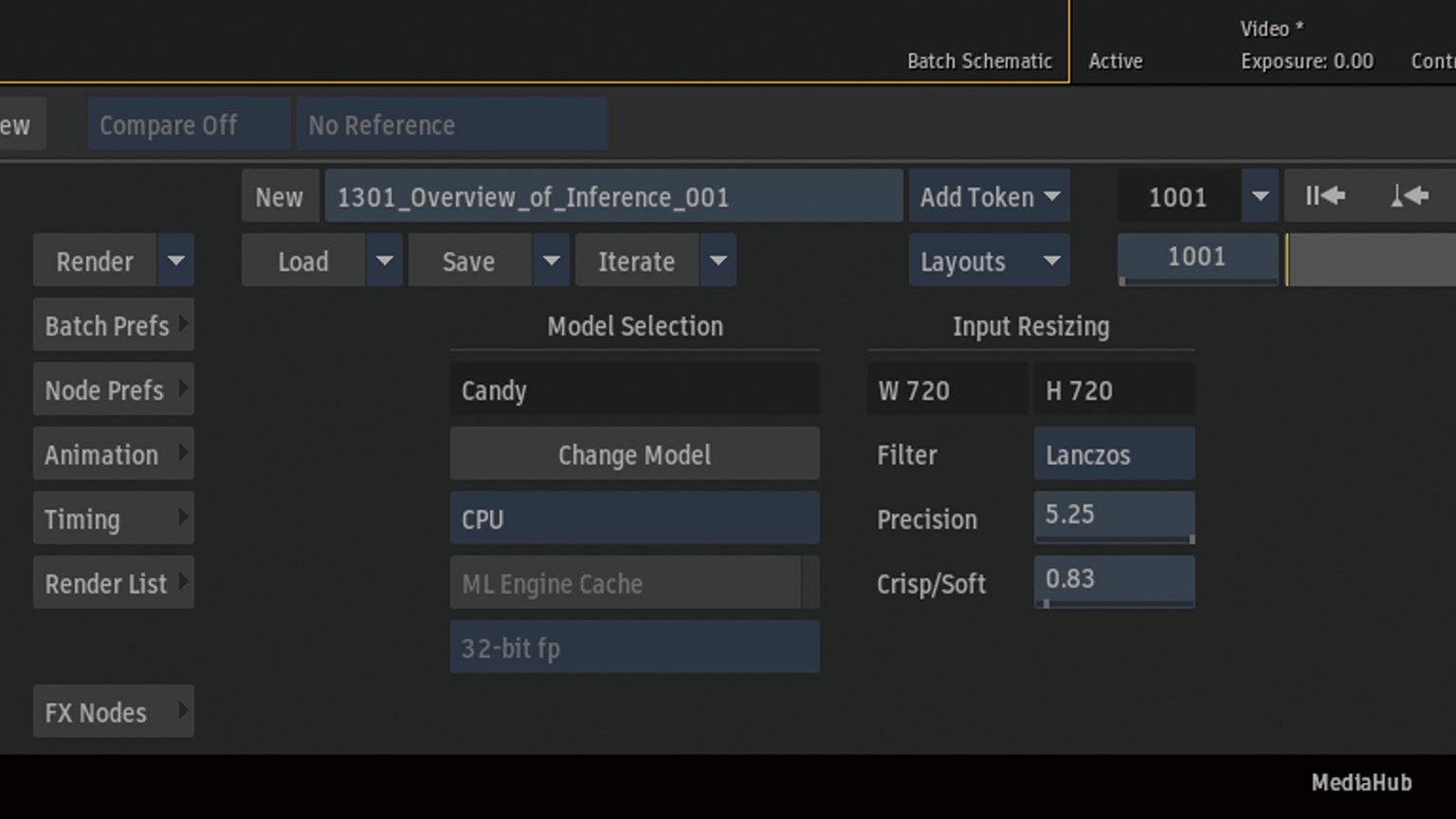
TensorRT
NVIDIAが提供するディープラーニング推論を最適化するツールです。学習済みのAIモデルをNVIDIA GPU上で高速かつ効率的に実行します。
キャッシュには、Machine Learning inferenceモデル(ML推論モデル)、GPU、および画像サイズの組み合わせごとに新しいTensorRTエンジンが必要になります。新しいモデルが新しい組み合わせに適用されるたびに新しいエンジンが生成されます。
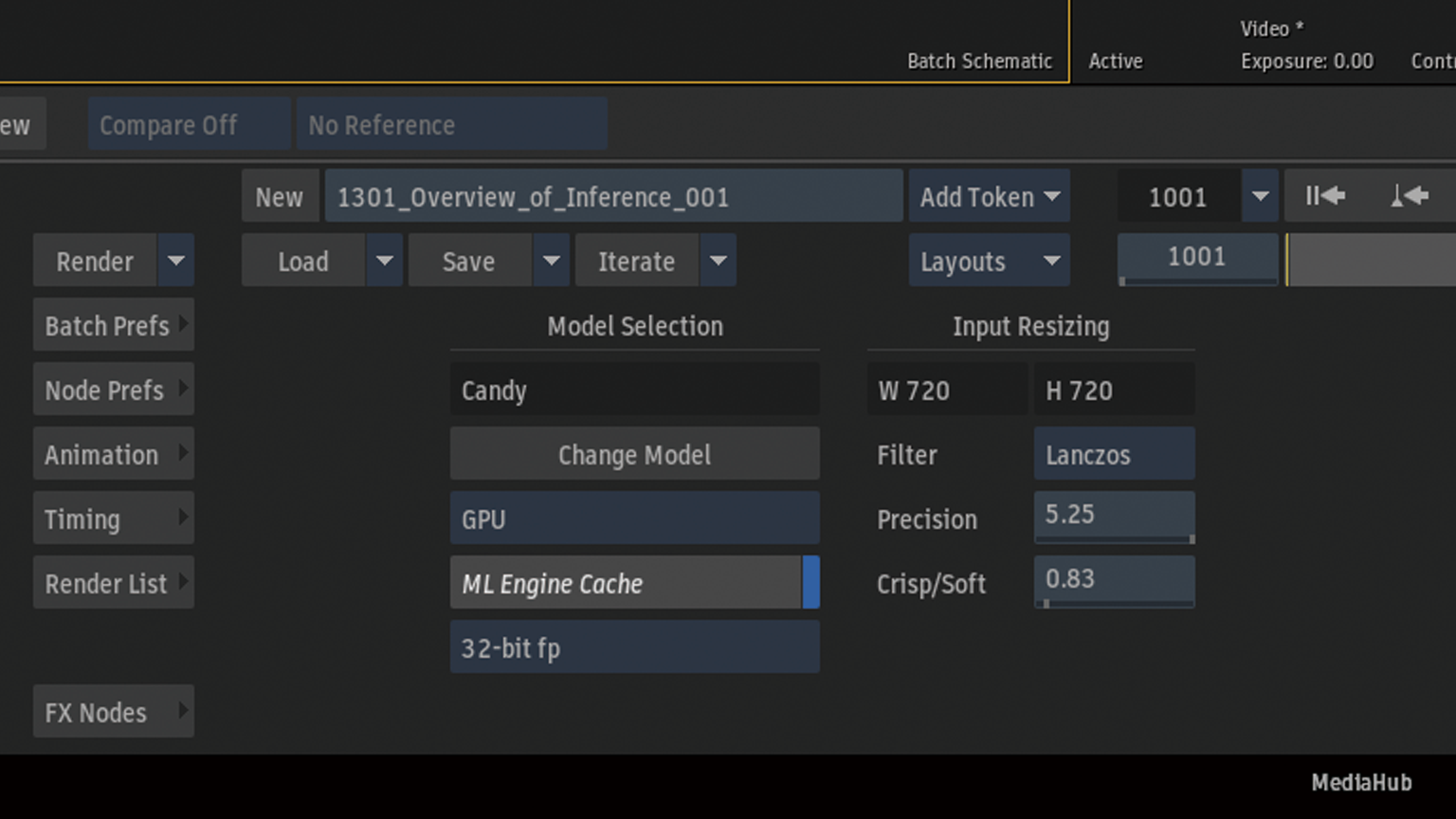
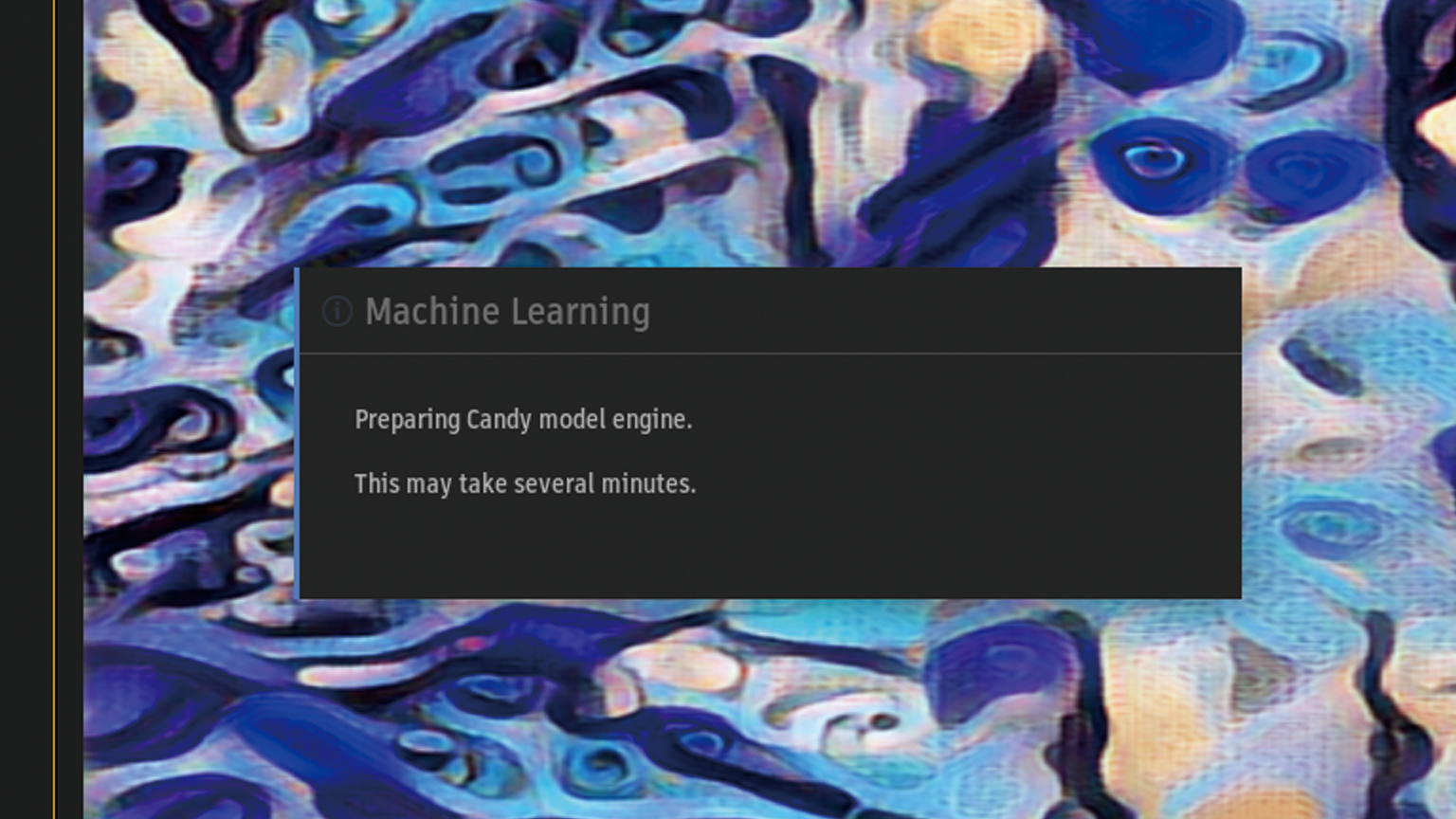
保存されたエンジンキャッシュは、同じML推論モデル、GPU、および画像サイズの組み合わせが必要になったときに使用されるため、大幅に高速化されます。
Cache > /opt/Autodesk/cache/tensorgraph/models